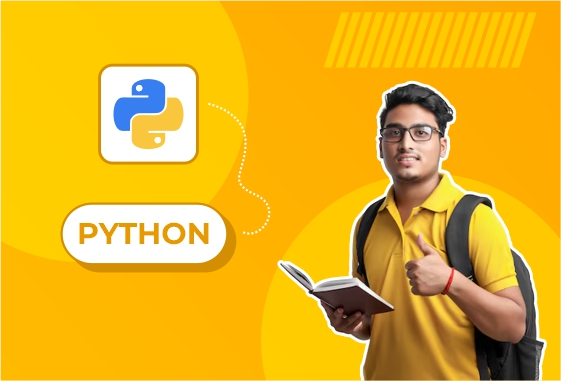
Handwritten Digit Recognition
Project Title: Handwritten Digit Recognition Using Machine Learning
Objective:
To build a model that can accurately recognize and classify digits (0–9) from handwritten images using machine learning or deep learning techniques.
Dataset:
MNIST Dataset: A widely used benchmark dataset containing 70,000 grayscale images (28x28 pixels) of handwritten digits.
Training Set: 60,000 images
Test Set: 10,000 images
Key Steps:
Data Preprocessing:
Normalize pixel values to a 0–1 range.
Reshape or flatten images (if using non-convolutional models).
Optionally apply data augmentation for improved generalization.
Model Building:
ML Models: Logistic Regression, SVM, k-NN (simpler approach).
Deep Learning: Convolutional Neural Networks (CNNs) for higher accuracy.
Training & Validation:
Split training data further into training and validation sets.
Use metrics like accuracy, precision, recall to evaluate model performance.
Testing:
Evaluate on the separate test set to measure generalization performance.
Results:
CNNs typically achieve >99% accuracy on the MNIST dataset.
Performance may vary depending on model complexity and training.
Tools & Libraries:
Python, NumPy, Pandas
Scikit-learn for ML models
TensorFlow or PyTorch for deep learning
Applications:
Postal code recognition
Bank check processing
Digit input in mobile devices