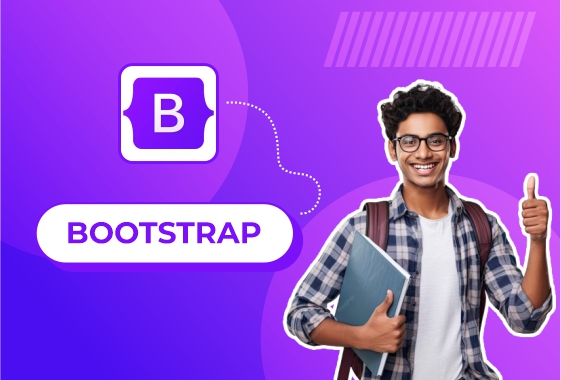
AI-powered product recommendations
Key Components of AI-Powered Recommendation Systems
Data Collection & Processing
AI gathers data from user interactions, purchase history, browsing behavior, and external sources.
It cleans and processes the data to detect patterns and trends.
Recommendation Algorithms
Collaborative Filtering – Suggests products based on similarities between users with shared interests.
Content-Based Filtering – Recommends products similar to those a user has previously interacted with.
Hybrid Models – Combines multiple recommendation techniques for improved accuracy.
Deep Learning & NLP – Uses advanced AI models, such as neural networks, to understand customer preferences.
Personalization Techniques
AI adapts recommendations based on user behavior in real-time.
Offers dynamic adjustments, seasonal suggestions, and location-based recommendations.
Context Awareness
AI takes into account time of day, trends, device type, and even sentiment analysis from reviews to enhance recommendations.
Benefits of AI-Powered Product Recommendations
✅ Enhanced User Experience – Personalized suggestions reduce search time and improve shopping satisfaction. ✅ Increased Conversion Rates – Well-targeted recommendations lead to higher engagement and sales. ✅ Customer Retention & Loyalty – AI fosters stronger relationships by making recommendations tailored to individual users. ✅ Optimized Inventory Management – Helps businesses understand product demand and stock availability. ✅ Cross-Selling & Upselling Opportunities – AI intelligently suggests complementary products to increase average order value.
Challenges & Considerations
⚠️ Data Privacy & Security – Ensuring ethical use of customer data while maintaining compliance with regulations like GDPR. ⚠️ Algorithm Bias – AI models must prevent biases in recommendations to ensure fairness. ⚠️ Cold Start Problem – New users or products may lack sufficient data for accurate recommendations. ⚠️ Over-Personalization – Excessive targeting may limit exposure to new products, reducing diversity in recommendations.
Real-World Applications & Examples
E-commerce Platforms: Amazon, Flipkart, Alibaba – AI suggests products based on user behavior.
Streaming Services: Netflix, Spotify, YouTube – Recommends content based on viewing and listening habits.
Retail & Fashion: Zalando, ASOS, Myntra – AI curates fashion choices based on style preferences.
Food & Grocery: Swiggy, Zomato, Instacart – AI predicts meal preferences and suggests food items.